AI News
About Digital Transformation by theme
If you are looking for something that you don't find in this section, but know it was here before, take a look at the archives section under the archives by theme tab
AI Documents
About Digital Transformation by theme
Insights from the "Today’s World Driven by Tomorrow’s Tech" Report
IE University’s Center for the Governance of Change (CGC) presents its latest Foresight report, "Today’s World Driven by Tomorrow’s Tech: Now, Next, New." As the world changes at an accelerating pace, understanding the trajectories of technological innovation has become crucial. This report, based on the Tech & Foresight Summit 2024, explores the key megatrends shaping our future and offers actionable insights for policymakers, businesses, and society.
The report identifies and explains nine vectors of change structured around the "Now, Next, New" framework:
Now:
Computing Divide – From connectivity to computing, an asset for a competitive and resilient economy.
Future of Money – Tracking financial innovation to anticipate socioeconomic change.
Individualized Societies – Redefining community in digitally individualized societies.
Next:
Data Centers – Can Europe scale its data centers securely, efficiently, and on time?
Sustainability of the Tech Sector – Finding the equilibrium between technological development and sustainability.
Humanizing Technology – Will technology serve humans or humanity?
New:
Defense Innovation – Can European industry keep up with the changing nature of war?
Quantum – Advancing quantum made in the EU.
Generational Inequalities – A growing polarization between age groups and generations.
The authors of this report are Irene Blázquez, Darío García de Viedma, Purvi Parekh and Giacco Ugarelli.
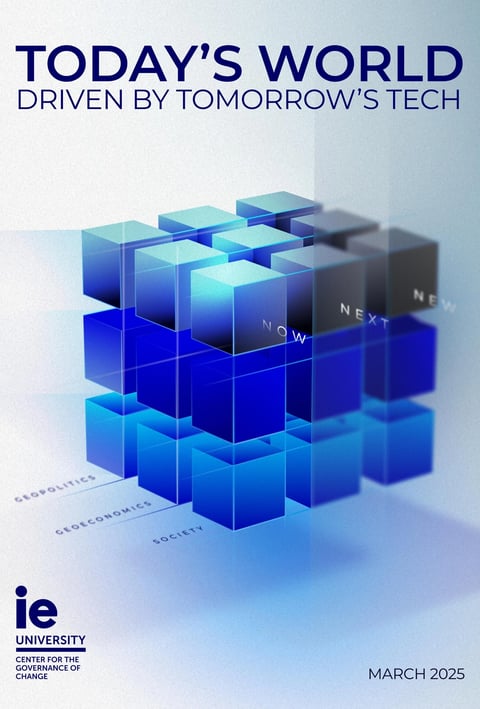
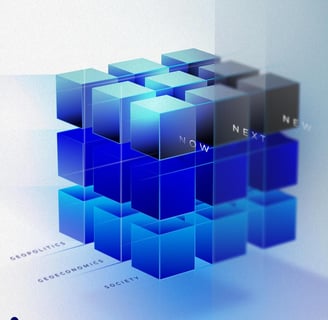
AI Documents
About Digital Transformation by theme
Julian de Freitas writes: Why people resist embracing AI
AI’s success hinges not only on its capabilities, which are becoming more advanced every day, but on people’s willingness to harness them. And as the Gartner findings suggest, AI is not getting great traction with users.
Unfortunately, most people are pessimistic about how it will shape the future. Seventy-seven percent of Americans are concerned that its adoption will cause job losses within the next 12 months, according to research by Forbes Advisor. Eighty percent think AI has increased the likelihood that their personal data will be used in malicious ways by criminals. And it gets worse: A poll conducted by YouGov found that nearly half of Americans believe that one day AI will attack humanity. With this much cynicism about AI, getting workers to willingly, eagerly, and thoroughly experiment with it is a daunting task.
In conducting more than a decade of research on adoption of the technology, including in-depth qualitative interviews and experiments with some 2,500 users, I have uncovered what’s driving this resistance to AI: fundamental human perceptions that AI is:
too opaque,
emotionless,
rigid, and independent, and
that interacting with humans is far more preferable.
Understanding those drivers is critical to designing interventions that will increase AI adoption inside organizations and among consumers generally. In this article I will delve into them in detail and explain what you can do as a manager to counter them.
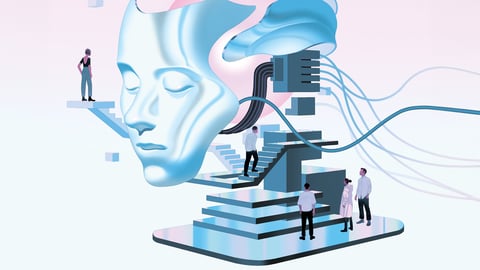
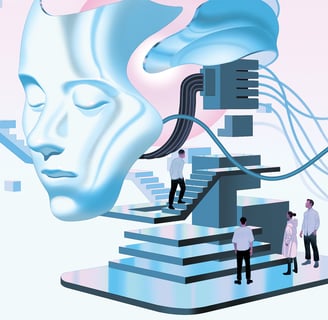
AI Documents
About Digital Transformation by theme
Timothy T Tiryaki writes: From VUCA to Flux
For nearly three decades, hashtag#VUCA (Volatile, Uncertain, Complex, Ambiguous) has shaped how we understand a world in transformation. Originally introduced in the book Leaders: Strategies for Taking Charge (1985), VUCA became a dominant framework for explaining the end of 20th century and the beginning of the 21st century.
But today, as AI rewrites the rules of business, leadership, and society, we must ask: Does VUCA still fully explain our reality?
We are entering an era where change is no longer just volatile—it’s constant and compounding. Uncertainty isn’t just something to anticipate—it’s embedded in the very fabric of AI-powered decision-making. Complexity has taken on new dimensions with AI’s ability to process vast amounts of data, and ambiguity has evolved into uncharted territories where traditional frameworks no longer apply.
In this new world, we need a new model—one that captures the speed, adaptability, and experimentation required to thrive in the AI era.
Welcome to the FLUX World
hashtag#FLUX represents a shift from merely surviving change to actively navigating and shaping the future. It is a framework designed for a world where AI, automation, and digital transformation are accelerating unpredictably.
FLUX – Fast, Liquid, Uncharted, eXperimental
🔵Fast – Change is no longer episodic; it’s continuous and compounding. Accelerated change or hashtag#transformation is one of the key pillars of this era. AI, automation, and emerging technologies are accelerating everything—from innovation cycles to decision-making. Leaders must move with agility, embracing real-time adaptation as a core competency.
🔵 Liquid – Traditional boundaries are dissolving. Industries are merging, business models and operating models are evolving and the old silos of business are breaking down. We are shifting from rigid structures to fluid ecosystems where collaboration, agility, and adaptability define success.
🔵 Uncharted – The AI era is rewriting the playbook. Organizations are stepping into unknown territories, where past data may not predict the future and traditional business models may no longer apply. In this world, foresight, strategic experimentation, and scenario planning become essential.
🔵 eXperimental – AI and emerging tech require an adaptive, test-and-learn mindset. Dealing with this level of complexity requires non-linear and probabilistic planning combined with rapid experimentation and high learning agility. Progress isn’t about finding the perfect answer—it’s about experimenting, learning, and adapting at speed. hashtag#rapidprototyping
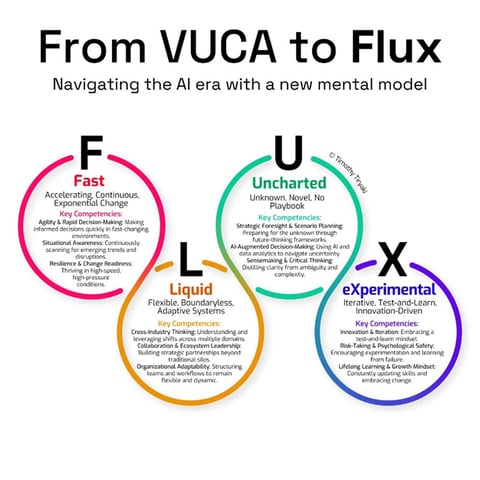
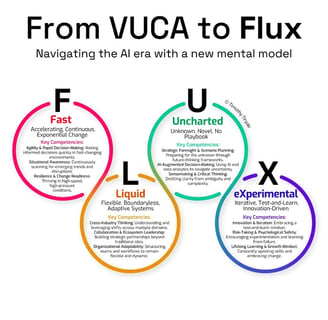
AI Documents
About Digital Transformation by theme
𝗪𝗲 𝗹𝗶𝘃𝗲 𝗶𝗻 𝗮 𝘄𝗼𝗿𝗹𝗱 𝗗𝗢𝗠𝗜𝗡𝗔𝗧𝗘𝗗 𝗮𝗻𝗱 𝗥𝗨𝗡 𝗯𝘆 9 𝗮𝗹𝗴𝗼𝗿𝗶𝘁𝗵𝗺𝘀!
The illustration below highlights the algorithms most frequently utilized in our everyday activities: They play a key role in everything we do from online shopping recommendations, navigation apps, social media, email spam filters and even smart home devices.
🔹 𝗦𝗼𝗿𝘁𝗶𝗻𝗴 𝗔𝗹𝗴𝗼𝗿𝗶𝘁𝗵𝗺
- Organize data for efficiency.
➜ Example: Sorting email threads or search results.
🔹 𝗗𝗶𝗷𝗸𝘀𝘁𝗿𝗮’𝘀 𝗔𝗹𝗴𝗼𝗿𝗶𝘁𝗵𝗺
- Finds the shortest path in networks.
➜ Example: Google Maps driving routes.
🔹 𝗧𝗿𝗮𝗻𝘀𝗳𝗼𝗿𝗺𝗲𝗿𝘀
- AI models that understand context and meaning.
➜ Example: ChatGPT, Claude and other LLMs.
🔹 𝗟𝗶𝗻𝗸 𝗔𝗻𝗮𝗹𝘆𝘀𝗶𝘀
- Ranks pages and builds connections.
➜ Example: TikTok PageRank, LinkedIn recommendations.
🔹 𝗥𝗦𝗔 𝗔𝗹𝗴𝗼𝗿𝗶𝘁𝗵𝗺
- Encrypts and secures data communication.
➜ Example: WhatsApp encryption or online banking.
🔹 𝗜𝗻𝘁𝗲𝗴𝗲𝗿 𝗙𝗮𝗰𝘁𝗼𝗿𝗶𝘇𝗮𝘁𝗶𝗼𝗻
- Secures cryptographic systems.
➜ Example: Protecting sensitive data in blockchain.
🔹 𝗖𝗼𝗻𝘃𝗼𝗹𝘂𝘁𝗶𝗼𝗻𝗮𝗹 𝗡𝗲𝘂𝗿𝗮𝗹 𝗡𝗲𝘁𝘄𝗼𝗿𝗸𝘀 (𝗖𝗡𝗡𝘀)
- Recognizes patterns in images and videos.
➜ Example: Facial recognition, object detection in self-driving cars.
🔹 𝗛𝘂𝗳𝗳𝗺𝗮𝗻 𝗖𝗼𝗱𝗶𝗻𝗴
- Compresses data efficiently.
➜ Example: JPEG and MP3 file compression.
🔹 𝗦𝗲𝗰𝘂𝗿𝗲 𝗛𝗮𝘀𝗵 𝗔𝗹𝗴𝗼𝗿𝗶𝘁𝗵𝗺 (𝗦𝗛𝗔)
- Ensures data integrity.
➜ Example: Password encryption, digital signatures.
Anything missing from your view?
---
Kudos to ByteByteGo for this amazing graphic!
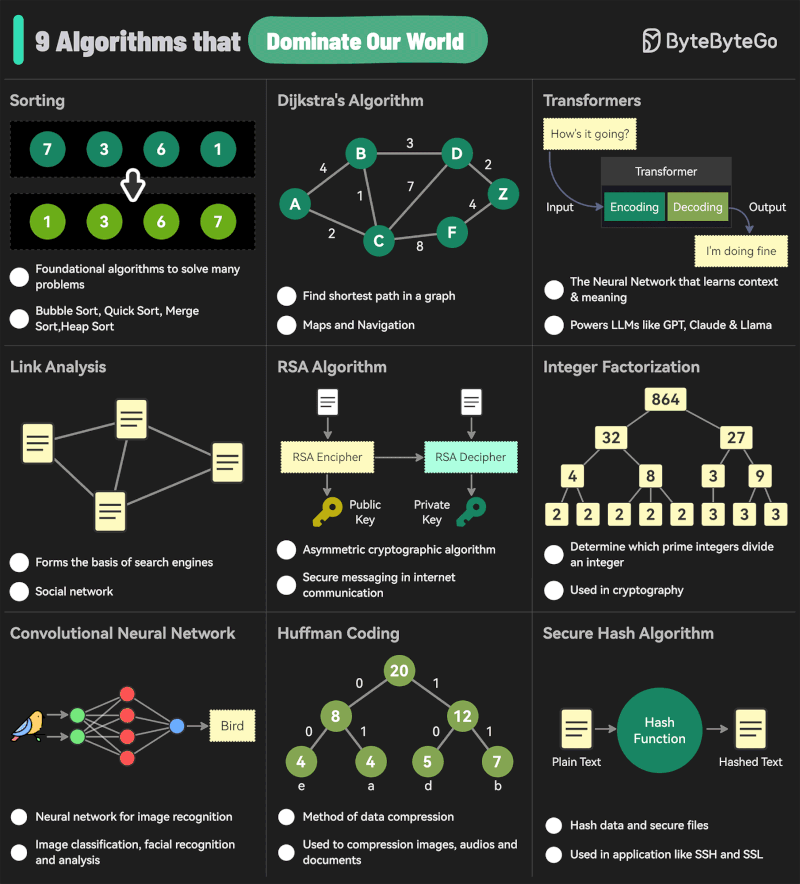
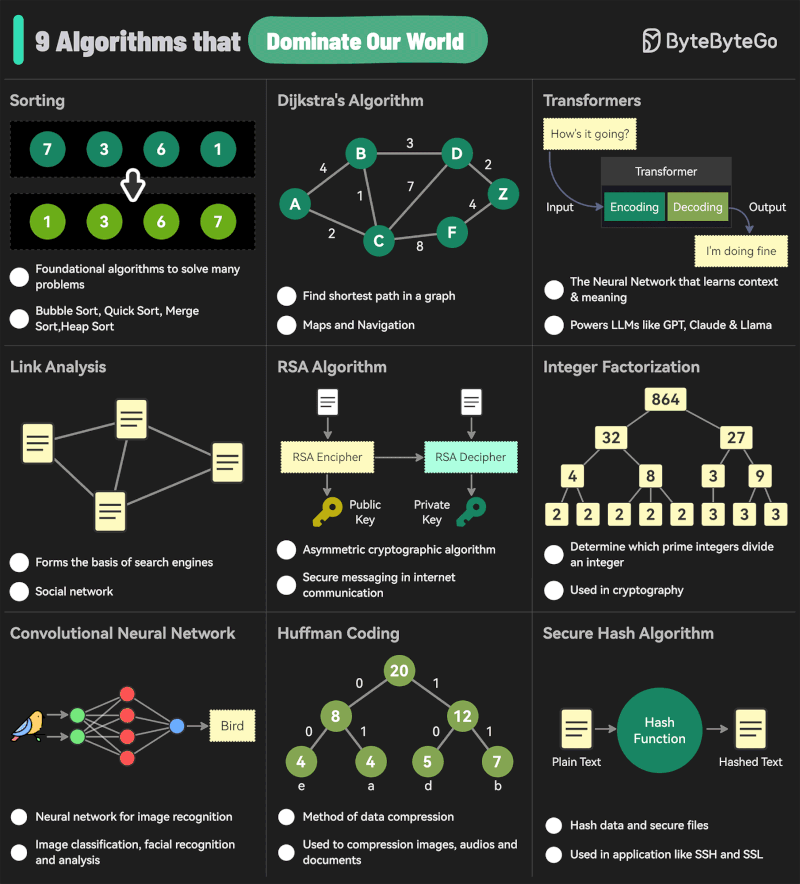
AI Tools
About Digital Transformation by theme
Government Digital Service
The UK government has today published its AI Playbook, a practical guide for civil servants and technical teams to responsibly develop, buy, and implement AI to improve public services.
With AI already helping to predict weather patterns, streamline MOT inspections, and supporting apprentices at risk of dropping out, the Playbook sets out 10 key principles to ensure AI is used transparently, effectively, and in the public interest.
💡 Some key takeaways:
🔹 AI should be used where it adds real value—not just for the sake of it.
🔹 Human oversight is essential to ensure AI supports, not replaces, decision-making.
🔹 Public sector teams should collaborate openly, sharing learnings and best practices.
This guidance builds on the AI Opportunities Action Plan, reinforcing the UK’s ambition to lead in AI innovation while improving efficiency across government.
📖 Read the AI Playbook: https://www.gov.uk/government/publications/ai-playbook-for-the-uk-government
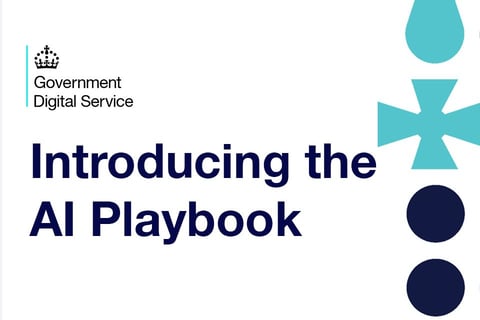
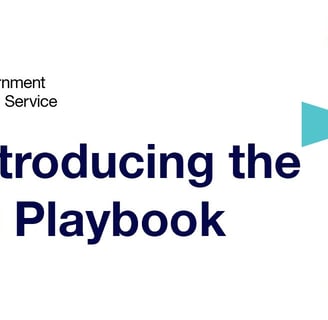
AI Tools
About Digital Transformation by theme
Marc Harris writes:
One of my most joyful discoveries last year was The Period Table of Evaluation created by Sara Vaca
When I shared the earlier version of the Periodic Table last Autumn it broke the field down into:
1️⃣ Paradigms
2️⃣ Purposes
3️⃣ Objectives
4️⃣ Criteria
5️⃣ Approaches
6️⃣ Designs
7️⃣ Methods
8️⃣ Frameworks
Here is the earlier version with the equally brilliant 'Catalogue of Evaluation Choices': https://lnkd.in/eH9gVgPa
The latest version offers a significant expansion now featuring 14 categories and 198 elements. Categories include:
1️⃣ Paradigms
2️⃣ Evaluation purposes
3️⃣ Findings uses
4️⃣ Process uses
5️⃣ Conceptualisation
6️⃣ Evaluator role
7️⃣ Evaluation questions
8️⃣ Sampling
9️⃣ Methods for data collections
🔟 Approaches to evaluation, analysis and reporting
"This Periodic Table of Evaluation (2025) is a collaborative effort between Michael (Q. Patton) and Sara (Vaca), created as a gift to the evaluation community. From June 2024 to January 2025, we’ve poured our passion and dedication into this project, holding countless discussions and working through more than 20 versions to get it right. Our hope is that this tool will inspire and support evaluators in their work, making complex concepts more accessible and practical for everyone."
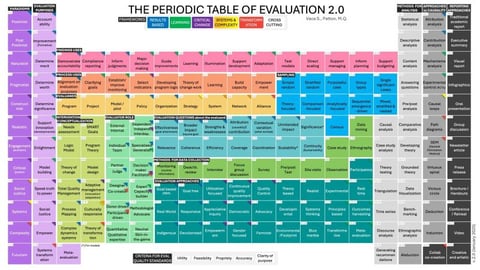
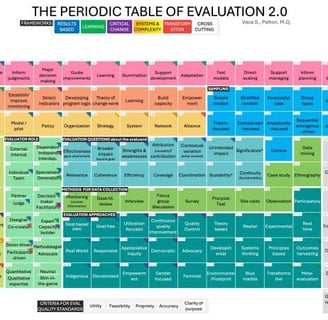
AI Tools
About Digital Transformation by theme
Brij kishore Pandey writes:
If you are into GenAI, you must know these 12 Terms -
1. LLM (Large Language Model)
- What: Advanced AI systems trained on massive text datasets
- Used in: ChatGPT, Claude, Gemini, Copilot
- Real-world example: GitHub Copilot using LLMs for code suggestions
2. Transformers
- What: Neural network architecture that revolutionized AI
- Used in: All modern language models (BERT, GPT, T5)
- Real-world example: Google BERT powering search results understanding
3. Prompt Engineering
- What: Art of crafting effective instructions for AI models
- Used in:
• Business applications
• Content creation
• Specialized tasks
- Real-world example: Writing prompts for image generation in DALL-E
4. Fine-tuning
- What: Customizing pre-trained models for specific tasks
- Used in:
• Industry-specific applications
• Custom chatbots
• Specialized tools
- Real-world example: Fine-tuning models for medical diagnosis
5. Embeddings
- What: Numerical representations of text/images
- Used in:
• Search engines
• Recommendation systems
• Document comparison
- Real-world example: Pinecone using embeddings for vector search
6. RAG (Retrieval Augmented Generation)
- What: Combining external knowledge with AI generation
- Used in:
• Enterprise chatbots
• Documentation tools
• Customer support
- Real-world example: Enterprise chatbots accessing company documentation
7. Tokens
- What: Units of text processing in AI models
- Used in:
• Model capacity planning
• Cost calculation
• Input/output management
- Real-world example: GPT-4's 32k token context window
8. Hallucination
- What: AI generating false but plausible information
- Impact on:
• Business applications
• Content generation
• Decision support
- Real-world example: ChatGPT generating incorrect historical dates
9. Zero-shot Learning
- What: AI performing tasks without specific training
- Used in:
• Classification tasks
• Language understanding
• New use cases
- Real-world example: Classifying new product categories without training
10. Chain-of-Thought
- What: Step-by-step reasoning in AI responses
- Used in:
• Problem solving
• Mathematical calculations
• Logic tasks
- Real-world example: Solving complex math problems step-by-step
11. Context Window
- What: Maximum text length AI can process
- Impact on:
• Document processing
• Conversation length
• Task complexity
- Real-world example: Processing long legal documents
12. Temperature
- What: Controls AI output randomness
- Used in:
• Creative writing
• Code generation
• Response variation
- Settings:
• Low (0.0): Consistent, focused
• High (0.7-1.0): Creative, varied
- Real-world example: Adjusting creativity in marketing copy generation
What other GenAI terms do you use regularly? Please share them below, and I will add them in the next iteration.
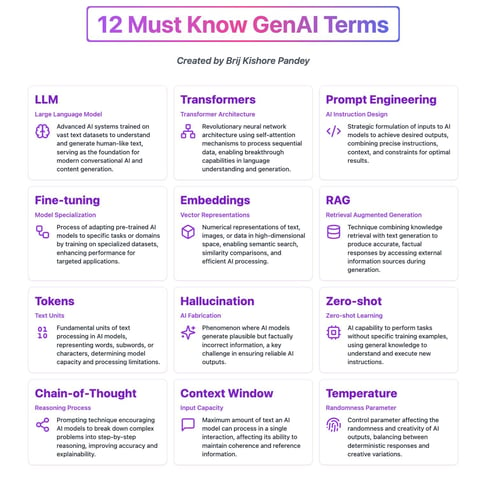
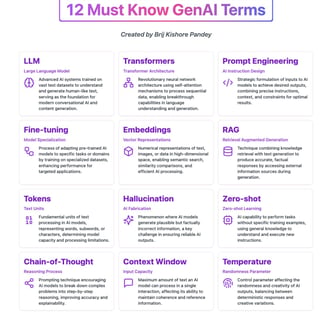
AI Tools
About Digital Transformation by theme
In Artificial Intelligence, Machine Learning, Data Science & Robotics Denis Panjuta writes:
Gen AI Mindmap Overview
🔹 Core Concepts – Includes transformers, neural networks, diffusion models, GANs, and RLHF, forming the foundation of generative AI.
🔹 Generative AI Models – Popular models like GPT, BERT, T5, DALL-E, and MidJourney power AI-driven creativity and automation.
🔹 Generative AI Tools – Platforms like ChatGPT, Bard, Claude, and ElevenLabs assist in content generation, coding, and automation.
🔹 Generative AI Applications – Covers text, image, video, and code generation, along with chatbots and data augmentation.
🔹 Popular Libraries & Frameworks – Essential tools like TensorFlow, PyTorch, LangChain, and Hugging Face streamline AI development.
🔹 Ethical & Security Concerns – Issues like AI bias, deepfakes, data privacy, and copyright highlight the need for responsible AI usage.
Generative AI is reshaping industries—stay ahead by understanding its key components!
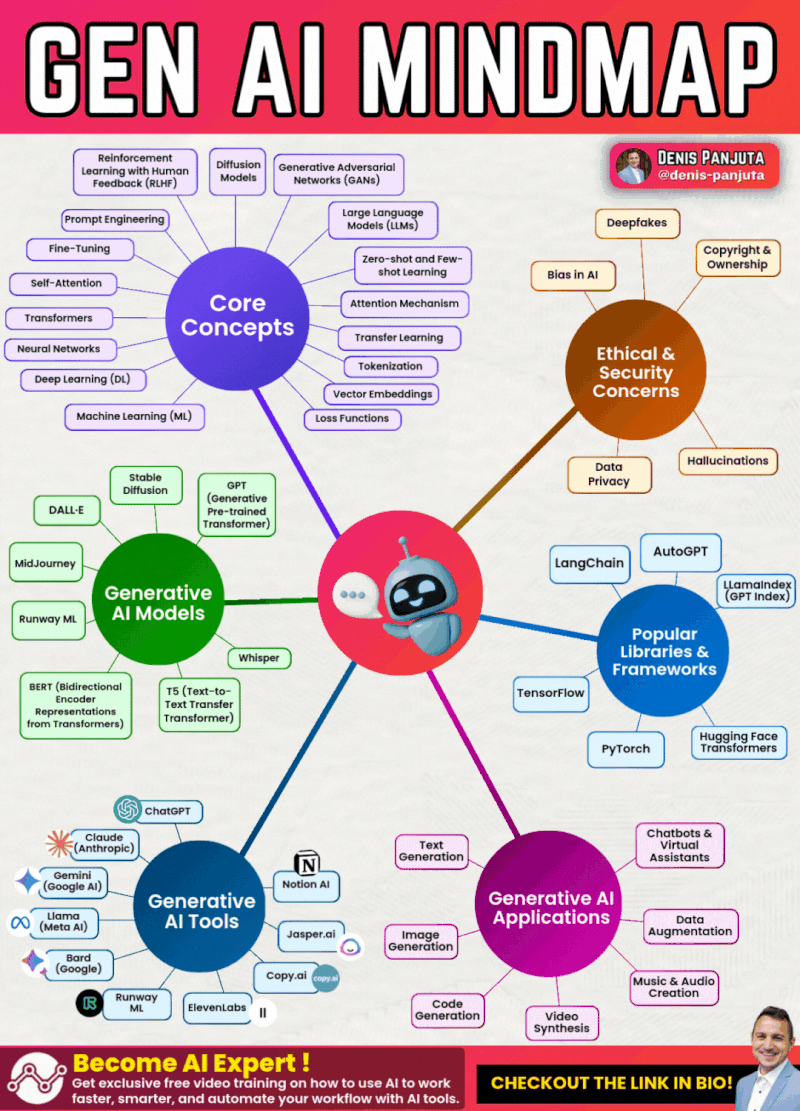
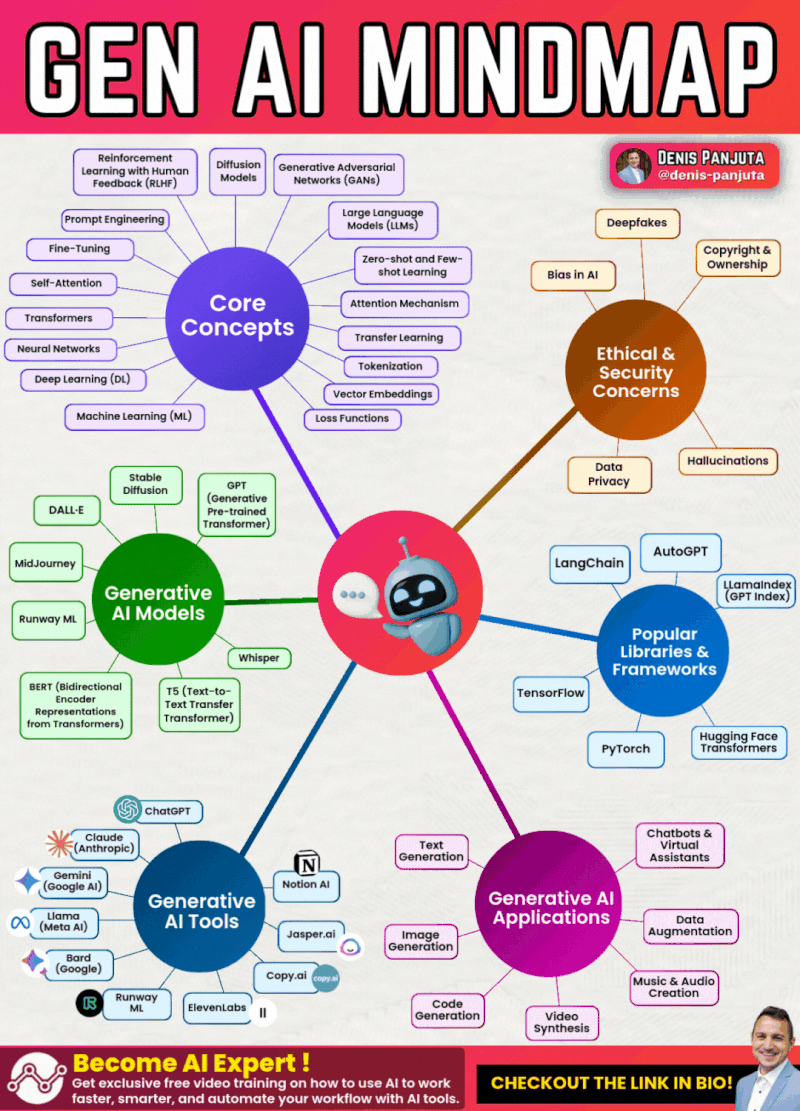
AI Tools
About Digital Transformation by theme
In Artificial Intelligence, Machine Learning, Data Science & Robotics
lori Dennehy writes:
𝐓𝐡𝐞 𝐀𝐈 𝐒𝐩𝐡𝐞𝐫𝐞: 𝐓𝐞𝐜𝐡𝐧𝐨𝐥𝐨𝐠𝐢𝐞𝐬 & 𝐁𝐞𝐧𝐞𝐟𝐢𝐭𝐬
𝐃𝐨𝐰𝐧𝐥𝐨𝐚𝐝 𝐩𝐝𝐟 𝐁𝐫𝐨𝐜𝐡𝐮𝐫𝐞 - https://lnkd.in/dxjpSNBx
🔵 𝐍𝐚𝐭𝐮𝐫𝐚𝐥 𝐋𝐚𝐧𝐠𝐮𝐚𝐠𝐞 𝐏𝐫𝐨𝐜𝐞𝐬𝐬𝐢𝐧𝐠 (𝐍𝐋𝐏)
Language Understanding
Speech Recognition
Language Generation
Syntax Parsing
Chatbots
Text-to-Speech (TTS)
Named Entity Recognition (NER)
Machine Translation
Sentiment Analysis
🔵 𝐂𝐨𝐦𝐩𝐮𝐭𝐞𝐫 𝐕𝐢𝐬𝐢𝐨𝐧
Image Recognition
Video Analysis
Image Generation
Object Detection
Style Transfer
Facial Recognition
Super-Resolution
Action Recognition
Object Tracking
Convolutional Neural Networks (CNNs)
🔵 𝐌𝐚𝐜𝐡𝐢𝐧𝐞 𝐋𝐞𝐚𝐫𝐧𝐢𝐧𝐠
Encompasses Supervised Learning
Reinforcement Learning
Unsupervised Learning
Ensemble Learning
Transfer Learning
Support Vector Machines (SVM)
Neural Networks
Policy Gradients
Clustering
🔵 𝐑𝐨𝐛𝐨𝐭𝐢𝐜𝐬
Features Autonomous Systems
Path Planning
Human-Robot Interaction
Gesture Recognition
Voice Commands
Simultaneous Localization and Mapping (SLAM)
Robotic Arms
Social Robots
Sensor Fusion
Grasping and Picking
🔵 𝐆𝐞𝐧𝐞𝐫𝐚𝐭𝐢𝐯𝐞 𝐀𝐈
Text Generation
Deepfakes
Image/Video Synthesis
Music Composition
Generative AI
Style Transfer
Synthetic Data Generation
Creative Writing
Poetry and Art Generation
AI-Composed Music
🔵 𝐊𝐧𝐨𝐰𝐥𝐞𝐝𝐠𝐞 𝐑𝐞𝐩𝐫𝐞𝐬𝐞𝐧𝐭𝐚𝐭𝐢𝐨𝐧 & 𝐑𝐞𝐚𝐬𝐨𝐧𝐢𝐧𝐠
Knowledge Graphs
Semantic Web
Ontologies
Logic and Inference
Probabilistic Reasoning
PlanningExpert Systems
Deductive Reasoning
Goal-Oriented Planning
Resource Allocation.
🔵𝐄𝐭𝐡𝐢𝐜𝐬 𝐚𝐧𝐝 𝐒𝐚𝐟𝐞𝐭𝐲 𝐢𝐧 𝐀𝐈
Accountability
Fairness
Safety,
Bias Detection
Algorithmic Transparency
Differential Privacy
Privacy
Responsible AI Development
Robustness to Adversarial Attacks
Legal and Regulatory Compliance.
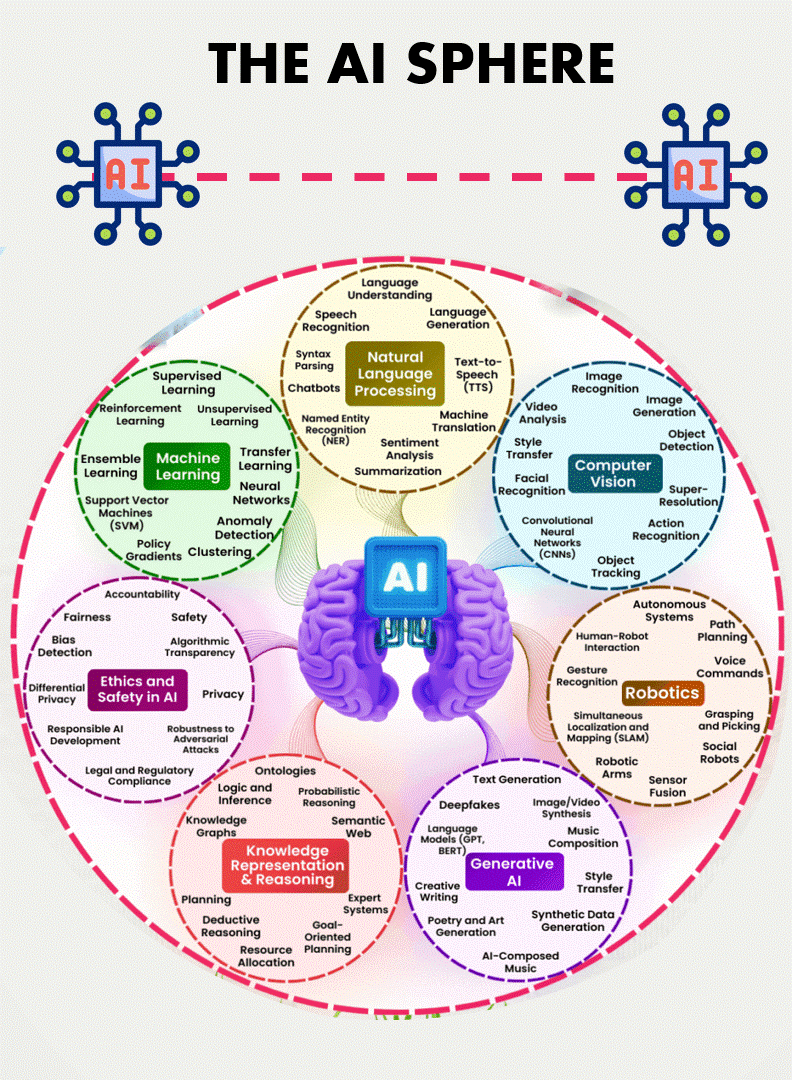
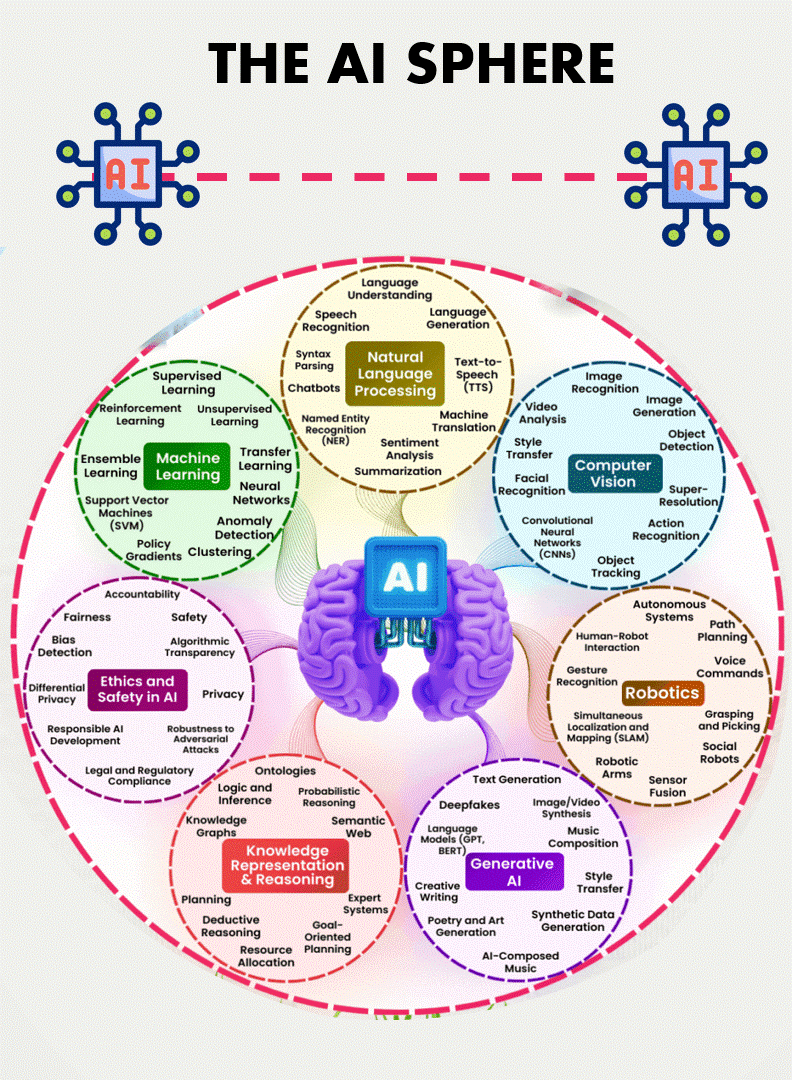
AI Tools
About Digital Transformation by theme
Peter Slattery, PhDPeter Slattery, PhD shares:
The Cambridge Handbook of the Law, Ethics and Policy of hashtag#AI has been released and is free to download (https://www.cambridge.org/core/books/cambridge-handbook-of-the-law-ethics-and-policy-of-artificial-intelligence/0AD007641DE27F837A3A16DBC0888DD1)
The book contains 20 Chapters (open access) covering topics on AI, ethics, philosophy, legal domains and sectoral applications.
Part I AI, Ethics and Philosophy
1 Artificial Intelligence: A Perspective from the Field
Wannes Meert, Tinne De Laet, and Luc De Raedt
2 Philosophy of AI: A Structured Overview
Vincent C. Müller
3 Ethics of AI: Toward a “Design for Values” Approach
Stefan Buijsman, Michael Klenk, and jeroen van den hoven
4 Fairness and Artificial Intelligence
Laurens Naudts and Anton Vedder
5 Moral Responsibility and Autonomous Technologies: Does AI Face a Responsibility Gap?
Lode Lauwaert and Ann-Katrien Oimann
6 Artificial Intelligence, Power and Sustainability
Gry Hasselbalch and Aimee van Wynsberghe
Part II AI, Law and Policy
7 AI Meets the GDPR: Navigating the Impact of Data Protection on AI Systems
Pierre Dewitte
8 Tort Liability and Artificial Intelligence: Some Challenges and (Regulatory) Responses
Jan De Bruyne and Wannes Ooms
9 Artificial Intelligence and Competition Law
Friso Bostoen
10 AI and Consumer Protection: An Introduction
Evelyne Terryn and Sylvia Martos Marquez
11 Artificial Intelligence and Intellectual Property Law
Jozefien Vanherpe
12 The European Union’s AI Act: Beyond Motherhood and Apple Pie?
Nathalie Smuha and Karen Yeung
Part III AI across Sectors
13 Artificial Intelligence and Education: Different Perceptions and Ethical Directions
Inge Molenaar, Duuk Baten, Imre Bárd, and Marthe Stevens 🟥
14 Artificial Intelligence and Media
Lidia Dutkiewicz, Noémie Krack, Aleksandra Kuczerawy, and Peggy Valcke
15 AI and Healthcare Data
Griet Verhenneman
16 Artificial Intelligence and Financial Services
Katja Langenbucher
17 Artificial Intelligence and Labor Law
Aida Ponce Del Castillo and Simon Taes
18 Legal, Ethical, and Social Issues of AI and Law Enforcement in Europe: The Case of Predictive Policing
Rosamunde Van Brakel
19 The Use of Algorithmic Systems by Public Administrations: Practices, Challenges and Governance Frameworks
Nathalie Smuha
20 Artificial Intelligence and Armed Conflicts
Katerina Yordanova
Thanks to Nathalie Smuha for sharing, and for her original post to promote this, which I have copied the above from.
AI History
About Digital Transformation by theme
Andreas Horn writes and shares the picture above:
𝗔𝘀 𝗔𝗜 𝗯𝗲𝗰𝗼𝗺𝗲𝘀 𝘀𝗼 𝗶𝗻𝗰𝗿𝗲𝗮𝘀𝗶𝗻𝗴𝗹𝘆 𝗽𝗼𝗽𝘂𝗹𝗮𝗿, 𝘄𝗲 𝘁𝗲𝗻𝗱 𝘁𝗼 𝗳𝗼𝗿𝗴𝗲𝘁 𝘁𝗵𝗲 𝗥𝗘𝗠𝗔𝗥𝗞𝗔𝗕𝗟𝗘 𝗽𝗿𝗼𝗰𝗲𝘀𝘀 𝗼𝘃𝗲𝗿 𝘁𝗵𝗲 𝗽𝗮𝘀𝘁 𝘀𝗲𝘃𝗲𝗿𝗮𝗹 𝗱𝗲𝗰𝗮𝗱𝗲𝘀! 📖
This infographic showcases the key milestones in AI history, highlighting its origins, evolution and the innovators who shaped it — from its early beginnings to today's transformative advancements.
🔹 1943: 𝗠𝗰𝗖𝘂𝗹𝗹𝗼𝗰𝗵 & 𝗣𝗶𝘁𝘁𝘀 create the first artificial neuron.
🔹 1950: 𝗔𝗹𝗮𝗻 𝗧𝘂𝗿𝗶𝗻𝗴 introduces the Turing Test, forever changing the way we view intelligence.
🔹 1956: 𝗝𝗼𝗵𝗻 𝗠𝗰𝗖𝗮𝗿𝘁𝗵𝘆 coins the term “Artificial Intelligence,” marking the official birth of the field.
🔹 1957: 𝗙𝗿𝗮𝗻𝗸 𝗥𝗼𝘀𝗲𝗻𝗯𝗹𝗮𝘁𝘁 invents the Perceptron, one of the first neural networks.
🔹 1959: 𝗕𝗲𝗿𝗻𝗮𝗿𝗱 𝗪𝗶𝗱𝗿𝗼𝘄 and 𝗧𝗲𝗱 𝗛𝗼𝗳𝗳 create ADALINE, a model that would shape neural networks.
🔹 1969: 𝗠𝗶𝗻𝘀𝗸𝘆 & 𝗣𝗮𝗽𝗲𝗿𝘁 solve the XOR problem, but also mark the beginning of the "first AI winter."
🔹 1980: 𝗞𝘂𝗻𝗶𝗵𝗶𝗸𝗼 𝗙𝘂𝗸𝘂𝘀𝗵𝗶𝗺𝗮 introduces Neocognitron, laying the groundwork for deep learning.
🔹 1986: 𝗚𝗲𝗼𝗳𝗳𝗿𝗲𝘆 𝗛𝗶𝗻𝘁𝗼𝗻 and 𝗗𝗮𝘃𝗶𝗱 𝗥𝘂𝗺𝗲𝗹𝗵𝗮𝗿𝘁 introduce backpropagation, making neural networks viable again.
🔹 1989: 𝗝𝘂𝗱𝗲𝗮 𝗣𝗲𝗮𝗿𝗹 advances UAT (Understanding and Reasoning), building a foundation for AI's logical abilities.
🔹 1995: 𝗩𝗹𝗮𝗱𝗶𝗺𝗶𝗿 𝗩𝗮𝗽𝗻𝗶𝗸 and 𝗖𝗼𝗿𝗶𝗻𝗻𝗮 𝗖𝗼𝗿𝘁𝗲𝘀 develop Support Vector Machines (SVMs), a breakthrough in machine learning.
🔹 1998: 𝗬𝗮𝗻𝗻 𝗟𝗲𝗖𝘂𝗻 popularizes Convolutional Neural Networks (CNNs), revolutionizing image recognition.
🔹 2006: 𝗚𝗲𝗼𝗳𝗳𝗿𝗲𝘆 𝗛𝗶𝗻𝘁𝗼𝗻 and 𝗥𝘂𝘀𝗹𝗮𝗻 𝗦𝗮𝗹𝗮𝗸𝗵𝘂𝘁𝗱𝗶𝗻𝗼𝘃 introduce deep belief networks, reigniting interest in deep learning.
🔹 2012: 𝗔𝗹𝗲𝘅 𝗞𝗿𝗶𝘇𝗵𝗲𝘃𝘀𝗸𝘆 and 𝗚𝗲𝗼𝗳𝗳𝗿𝗲𝘆 𝗛𝗶𝗻𝘁𝗼𝗻 launch AlexNet, sparking the modern AI revolution in deep learning.
🔹 2014: 𝗜𝗮𝗻 𝗚𝗼𝗼𝗱𝗳𝗲𝗹𝗹𝗼𝘄 introduces Generative Adversarial Networks (GANs), opening new doors for AI creativity.
🔹 2017: 𝗔𝘀𝗵𝗶𝘀𝗵 𝗩𝗮𝘀𝘄𝗮𝗻𝗶 and team introduce Transformers, redefining natural language processing (NLP).
🔹 2020: OpenAI unveils GPT-3, setting a new standard for language models and AI’s capabilities.
🔹 2022: OpenAI releases ChatGPT, democratizing conversational AI and bringing it to the masses.
𝗦𝗼, 𝗶𝘁 𝘁𝗼𝗼𝗸 𝗱𝗲𝗰𝗮𝗱𝗲𝘀 𝗼𝗳 𝘁𝗿𝗶𝗮𝗹, 𝗲𝗿𝗿𝗼𝗿 𝗮𝗻𝗱 𝗶𝗻𝗻𝗼𝘃𝗮𝘁𝗶𝗼𝗻 𝘁𝗼 𝗴𝗲𝘁 𝘂𝘀 𝘁𝗼 𝘄𝗵𝗲𝗿𝗲 𝘄𝗲 𝗮𝗿𝗲 𝘁𝗼𝗱𝗮𝘆, 𝘄𝗶𝘁𝗵 𝘁𝘄𝗼 𝗵𝘆𝗽𝗲 𝗰𝘆𝗰𝗹𝗲𝘀 𝗮𝗹𝗼𝗻𝗴 𝘁𝗵𝗲 𝘄𝗮𝘆. 𝗜𝘁'𝘀 𝗮𝗻 𝗲𝘅𝗰𝗶𝘁𝗶𝗻𝗴 𝗮𝗻𝗱 𝘁𝗿𝗮𝗻𝘀𝗳𝗼𝗿𝗺𝗮𝘁𝗶𝘃𝗲 𝘁𝗶𝗺𝗲 𝗶𝗻 𝗵𝘂𝗺𝗮𝗻 𝗵𝗶𝘀𝘁𝗼𝗿𝘆 𝗮𝗻𝗱 𝘄𝗲'𝗿𝗲 𝘃𝗲𝗿𝘆 𝗳𝗼𝗿𝘁𝘂𝗻𝗮𝘁𝗲 𝘁𝗼 𝘄𝗶𝘁𝗻𝗲𝘀𝘀 𝗶𝘁 𝘂𝗻𝗳𝗼𝗹𝗱 𝗿𝗶𝗴𝗵𝘁 𝗶𝗻 𝗳𝗿𝗼𝗻𝘁 𝗼𝗳 𝘂𝘀!
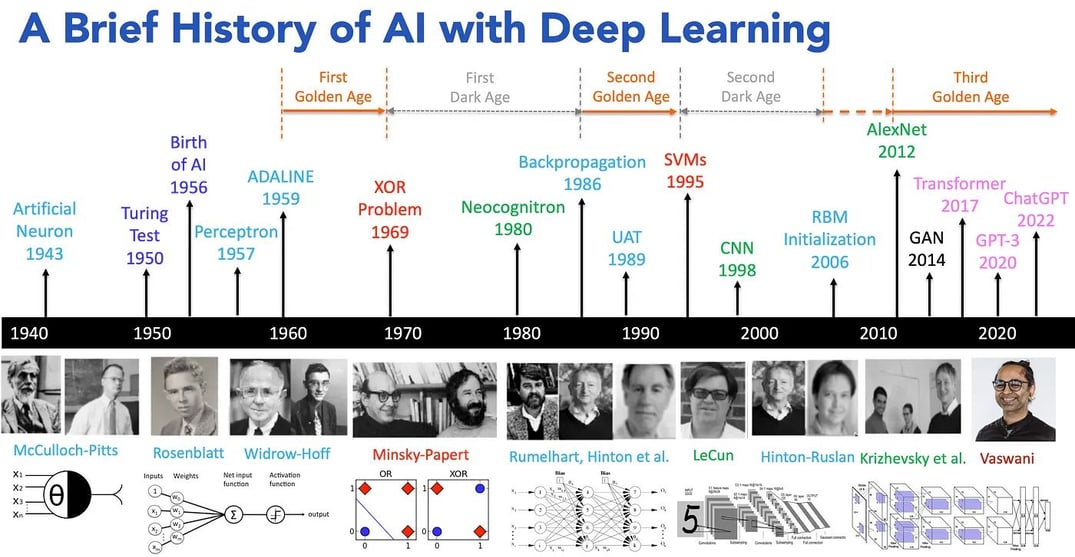
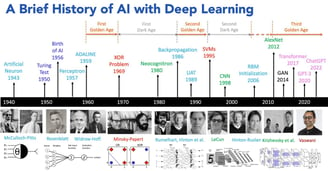
AI Documents
About Digital Transformation by theme
World Economic Forum writes:
DeepSeek AI has shaken up the #AI industry, seemingly demonstrating it’s possible to build models faster and cheaper than previously thought.
But what is the impact of making its code free for all? And how could open source AI democratize the #technology?
Learn more: https://www.weforum.org/stories/2025/02/open-source-ai-innovation-deepseek/
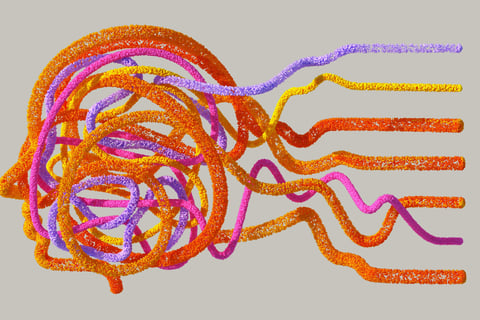
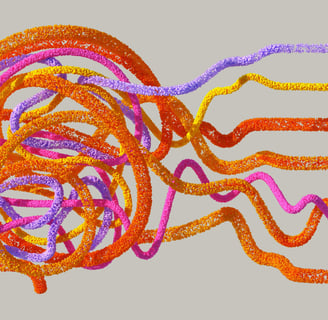
AI Tools
About Digital Transformation by theme
Federico Marengo posted the AI Playbook for the UK Government
Yesterday UK Gov launched the Playbook for the UK Government to provide departments and public sector organisations with accessible technical guidance on the safe and effective use of AI.
Critical for civil servants to gain an understanding of what AI can and cannot do, how it can help, and the potential ethical, legal, privacy, sustainability and security risks it poses.
What I find interesting
How to create the AI support structure (p37)
- AI strategy and adoption plan
- AI principles
- AI governance board
- AI comms strategy
- AI sourcing and partnership strategy
- AI training
How to deploy AI securely (p74-77)
Critical to understand the different ways AI can be found the in the organization
- public AI applications and web services (ChatGPT, Claude)
- embedded AI applications (Copilot)
- public AI APIs (eg OpenAI)
- privately hosted AI models (own private cloud infrastructure)
- Managed ML model hosting platform (AWS Bedrock, Azure)
- running AI models locally (eg on device)
- working with your organisational data (fine tune or RAG)
AI Tools
About Digital Transformation by theme
Stuart MacKinnon just wrote:
The NZ Public Service AI Framework and Guidelines just dropped. Here are some quick thoughts – keen to hear yours.
✅ What’s welcome
• The "light-touch, proportionate, and risk-based" approach suggests a balanced, pragmatic stance.
• Reinforces that privacy, security, and effective government remain non-negotiables.
🔍 What I'd like to see evolve
• AI covers everything from simple office automation to more complex use cases – each with different risks. Future 'worked examples' will strengthen guidance.
• The current web format feels a little fragmented. Be sure to click on "+" symbols to read all content.
💡 Potential challenges for agencies
• AI will put pressure on existing risk, assurance, and governance processes – now is the time to review readiness.
• Transparency will require uncompromising clarity – from concept to build, testing, and evaluation.
• Assuming AI is “too hard” might lead to missed opportunities. The right tech, safely implemented, can deliver value.
• Finding skilled, independent advice isn’t always easy – reach out anytime if I can help.
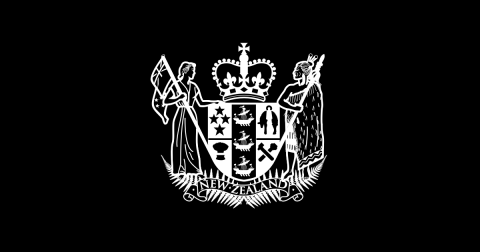
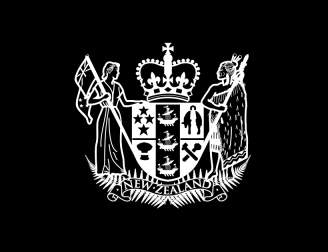
AI Training
About Digital Transformation by theme
David Panjuta presents please see his profile for full text
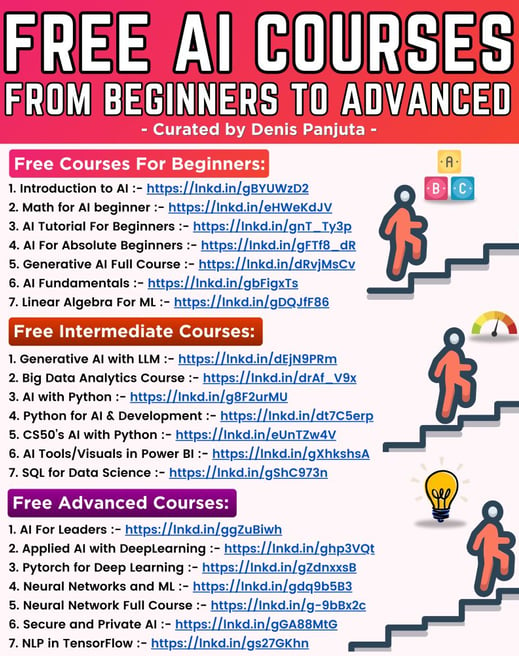
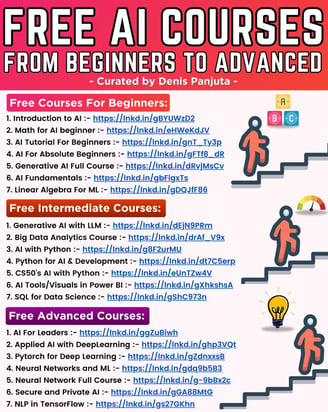
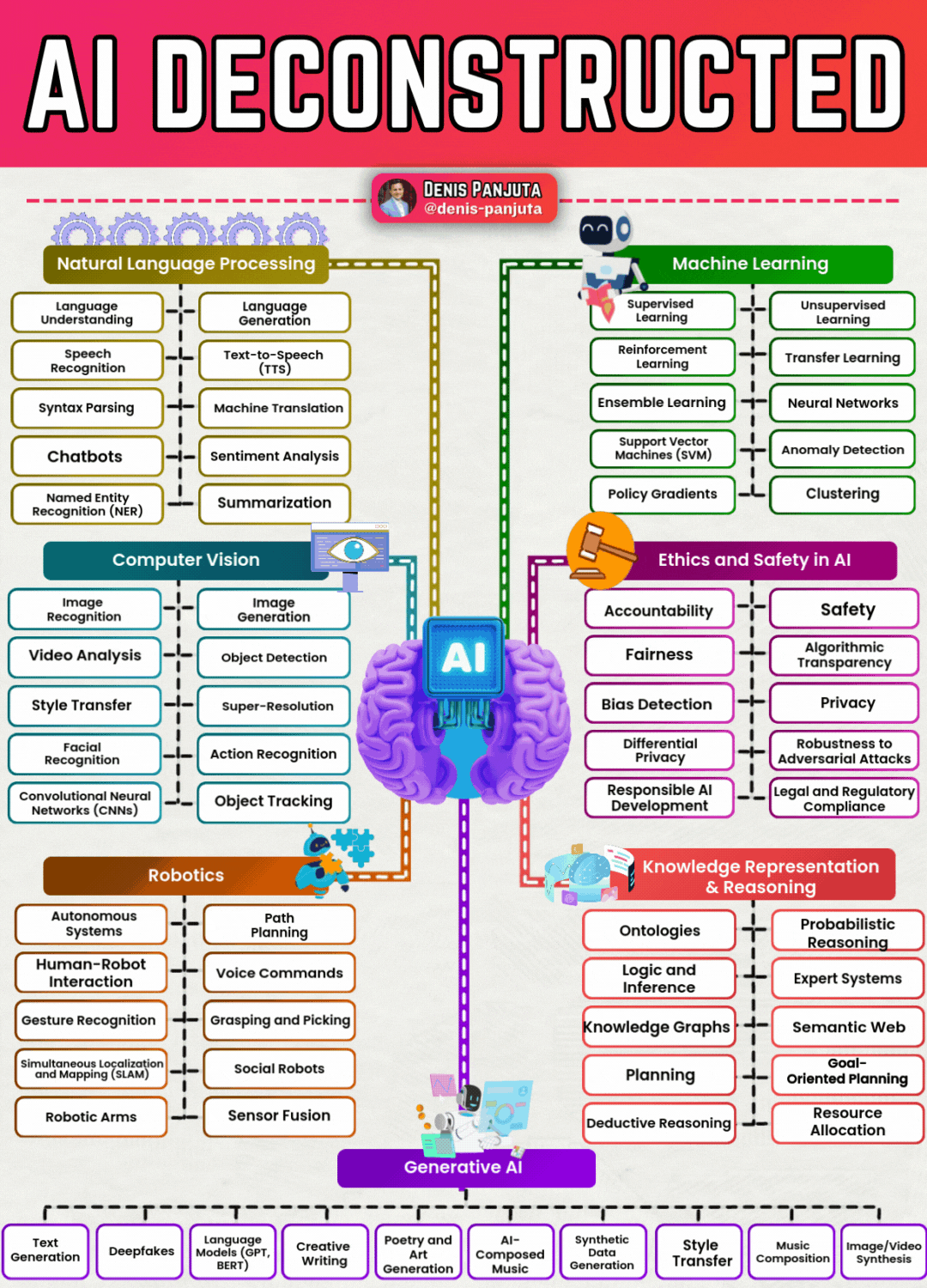
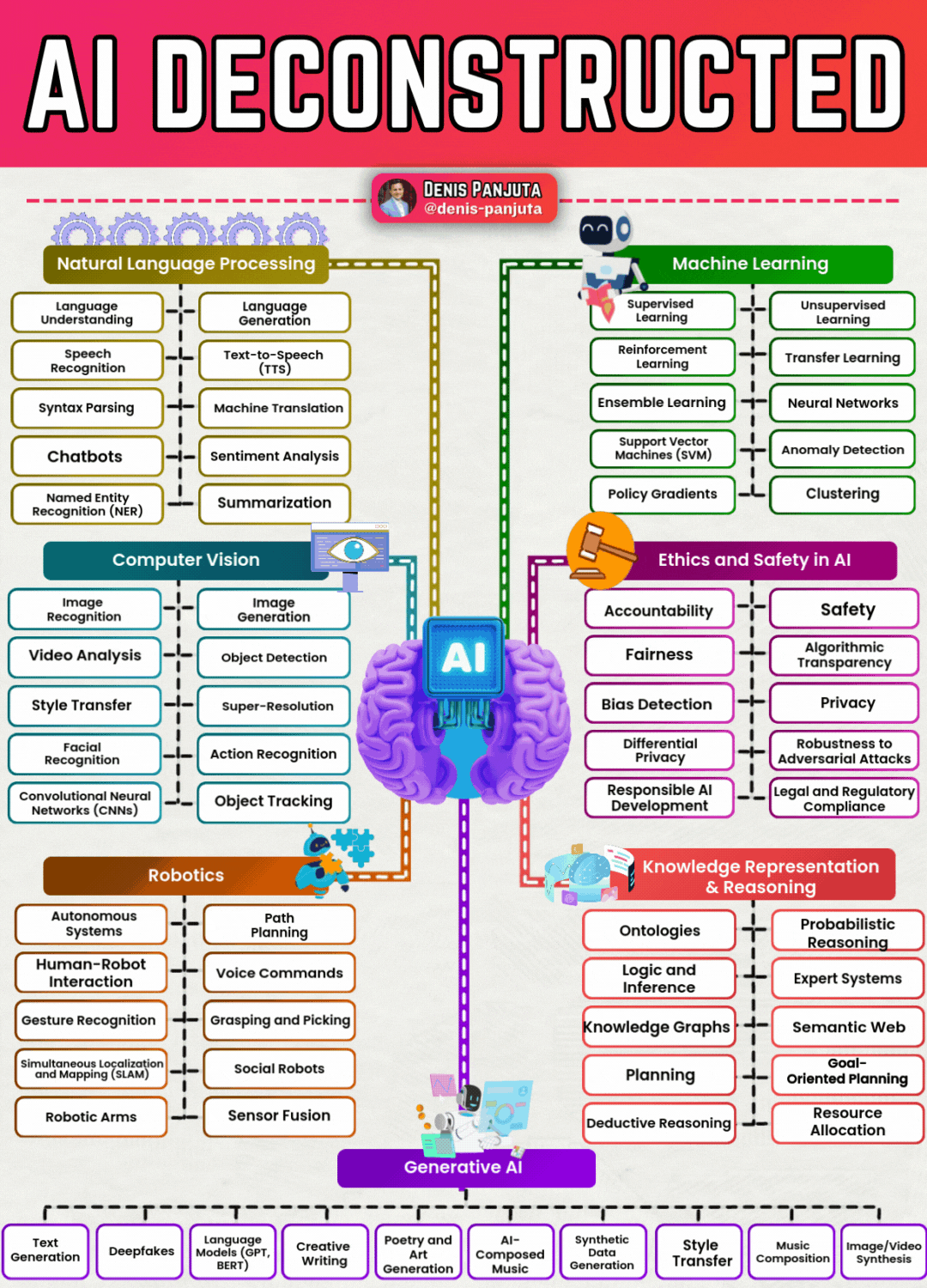
Tigran Egiazarov shares:
AI-powered intelligent applications and systems are the Future of Enterprise Applications. They intelligently adapt to business processes, user input, and user output. AI agents, LLM-powered applications, combined with AI-ready data and discoverable APIs, provide the perfect set of ingredients to start enhancing your enterprise applications.
With recent developments and cost reductions in the LLM area (though it can't yet be called a commodity), organizations should focus on business applications and customer experience, assuming that the cost of LLMs will continue to drop. Therefore, buy LLMs, use RAG, fine-tune if necessary, distill them, but concentrate on the level where "blending" will occur. LLMs may come and go, but your connected AI-ready data and API-enabled applications will remain to support any AI agent technologies.
Akis Sklavounakis, Tad Travis and Anne Thomas recently published a research "CIO’s Decision Guide: Buy, Build, Insource or Outsource Applications" https://lnkd.in/dcSvhES3. Congratulations!
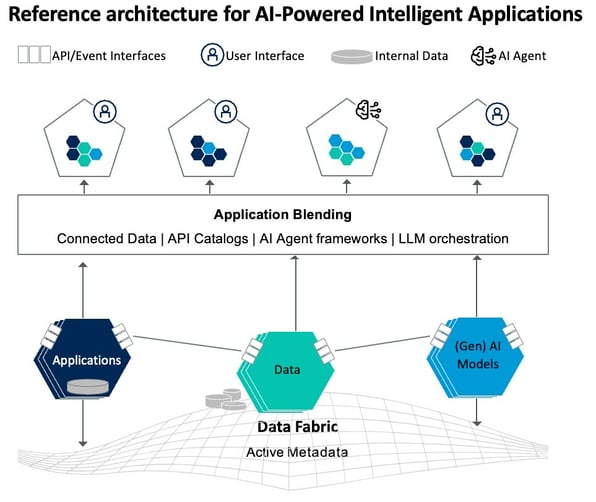
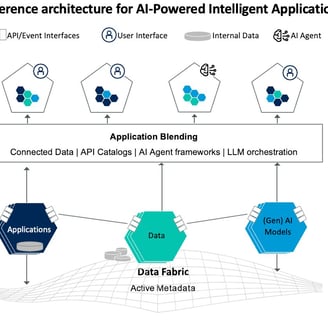
Armand Ruiz explains:
AI Agents 101 - Let's learn the basics:
𝗪𝗵𝗮𝘁 𝗜𝘀 𝗮𝗻 𝗔𝗴𝗲𝗻𝘁?
An AI agent is a system designed to reason through complex problems, create actionable plans, and execute these plans using a suite of tools.
It follows this continuous cycle:
1/ Think – The agent processes available data and context.
2/ Plan – It decides on a strategy to achieve a goal or answer a question.
3/ Act – It executes the chosen plan (e.g., makes an API call, retrieves data, or interfaces with a user).
4/ Reflect – It evaluates the outcome of its actions, checks for errors or new insights, and uses those reflections to inform the next iteration of thought.
These four steps form a feedback loop that enables agents to adapt their approach as new information arrives.
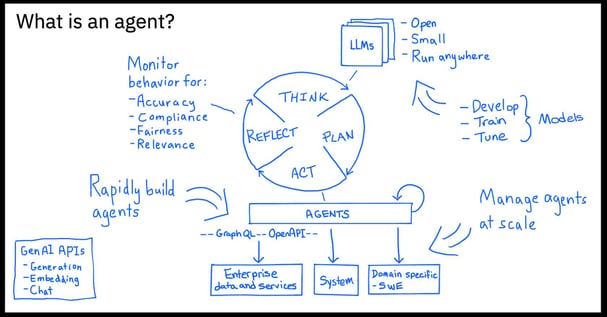
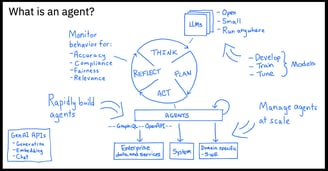
Armand Ruiz continues:
𝗧𝗵𝗲 𝗥𝗼𝗹𝗲 𝗼𝗳 𝗟𝗟𝗠𝘀
Under the hood, agents leverage LLMs and their brains. These LLMs can be:
1/ Open: Harnessing publicly available models or open-source alternatives.
2/ Small: Optimized or compressed versions that can run on local hardware more efficiently.
3/ Trained & Tuned Anywhere: Thanks to modern tooling, LLMs can now be developed, fine-tuned, and deployed in various environments—from the public cloud to on-premises servers.
LLMs provide the core reasoning, comprehension, and language generation abilities that agents require.
𝗛𝗼𝘄 𝗔𝗜 𝗔𝗴𝗲𝗻𝘁𝘀 𝗖𝗼𝗺𝗽𝗹𝗲𝘁𝗲 𝗧𝗮𝘀𝗸𝘀
Putting everything together, the workflow of an AI agent system might look like this:
1/ User or system triggers a request.
2/ LLM-based agent uses an internal reasoning loop (think → plan → act → reflect) to interpret the request and plan next steps.
3/ The agent makes calls to GenAI APIs or enterprise data/services (via GraphQL, OpenAPI, etc.) to gather more information.
4/ The agent processes the gathered data and formulates a response or an action.
5/ It executes that action or generates a final output to the user or system.
6/ Logging and monitoring systems record metrics (accuracy, compliance, fairness, relevance) so that developers and administrators can refine the agent’s behavior.
The business world is not yet ready to fully adopt agents; they are still in the very early experimental stage, and that's why everyone is implementing RAG like crazy, as it is a safer bet.
But believe me, AI agents are where it's at, and at the current pace of innovation, we will soon start collaborating with AI agents instead of humans for some specific tasks.
AI Tools
About Digital Transformation by theme